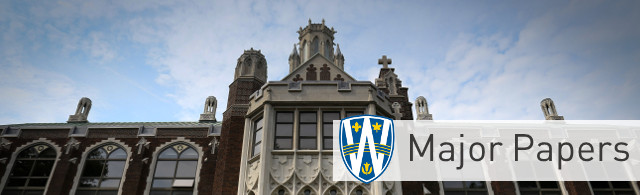
Keywords
missing value, variable selection, missForest, self-training selection, random lasso, Meta-analysis
Abstract
Modern Statistics has entered the era of Big Data, wherein data sets are too large, high-dimensional, incomplete and complex for most classical statistical methods. This analysis of Big data firstly focuses on missing data. We compare different multiple imputation methods. Combining the characteristics of medical high-throughput experiments, we compared multivariate imputation by chained equations (MICE), missing forest (missForest), as well as self-training selection (STS) methods. A phenotypic data set of common lung disease was assessed. Moreover, in terms of improving the interpretability and predictability of the model, variable selection plays a pivotal role in the following analysis. Taking the Lasso-Poisson model as an example, we illustrate the robust random Lasso method in the Meta-analysis of multiple datasets for variable selection. Thus, the real data analysis clarifies that missForest and STS outperform MICE. Moreover, the simulation results show that although this method is as effective in selecting important variables as using the random Lasso method, meta-analysis based on the random Lasso is better in terms of coefficient estimation and elimination of unimportant variables. In conclusion, We firstly propose a missForest random lasso (MFRL) method to complete the multiple imputation of the high-dimensional data and robustly select important variables.
Primary Advisor
Sévérien Nkurunziza
Program Reader
Abdul A. Hussein
Degree Name
Master of Science
Department
Mathematics and Statistics
Document Type
Major Research Paper
Convocation Year
2020