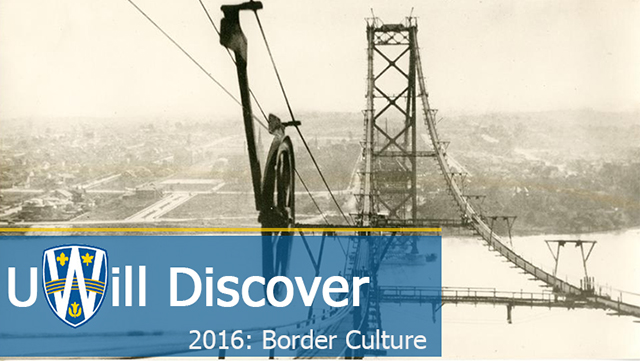
Identifying Novel Biomarkers for Monitoring Prostate Cancer Progression Using RNA-Seq data and Machine Learning Techniques
Type of Proposal
Oral presentation
Faculty
Faculty of Science
Faculty Sponsor
Dr. Lisa Porter
Proposal
Prostate cancer is the most frequently diagnosed cancer among Canadian men, with 1 in every 8 men diagnosed in their lifetime. Although prognosis at early stages is favourable, distinguishing which prostate cancer cases are at high-risk of progressing to invasive carcinoma remains a major challenge. As such, many prostate cancer patients who are at low-risk of progression are being unnecessarily subjected to invasive and risky treatments (i.e., surgery). As part of a collaboration with researchers from computer science, we will test select RNA splice variants implicated as being differentially expressed in different stages of prostate cancer. Our computer science partners used machine learning techniques, a method of using computer algorithms to recognize and analyze patterns within a dataset, to identify prostate stage-specific differences in RNA transcripts. Of the set of RNA transcripts identified, we have verified that transcripts for two genes – WWP2 and STEAP2– are upregulated at the RNA and protein level in the prostate cancer cell lines DU145 and LNCaP. Using qPCR techniques, we are currently validating expression of these transcripts in a series of WPE1 prostate cancer cell lines that mimic prostate cancer progression (i.e. benign, dysplastic, and invasive). We are also beginning to evaluate the mechanisms by which these transcripts are involved in prostate cancer progression. Identification of these genes and their transcripts as potential biomarkers of disease progression may lead to the development of novel diagnostic tools and less invasive and more efficacious targeted therapies.
Start Date
29-3-2016 1:00 PM
End Date
29-3-2016 2:20 PM
Identifying Novel Biomarkers for Monitoring Prostate Cancer Progression Using RNA-Seq data and Machine Learning Techniques
Prostate cancer is the most frequently diagnosed cancer among Canadian men, with 1 in every 8 men diagnosed in their lifetime. Although prognosis at early stages is favourable, distinguishing which prostate cancer cases are at high-risk of progressing to invasive carcinoma remains a major challenge. As such, many prostate cancer patients who are at low-risk of progression are being unnecessarily subjected to invasive and risky treatments (i.e., surgery). As part of a collaboration with researchers from computer science, we will test select RNA splice variants implicated as being differentially expressed in different stages of prostate cancer. Our computer science partners used machine learning techniques, a method of using computer algorithms to recognize and analyze patterns within a dataset, to identify prostate stage-specific differences in RNA transcripts. Of the set of RNA transcripts identified, we have verified that transcripts for two genes – WWP2 and STEAP2– are upregulated at the RNA and protein level in the prostate cancer cell lines DU145 and LNCaP. Using qPCR techniques, we are currently validating expression of these transcripts in a series of WPE1 prostate cancer cell lines that mimic prostate cancer progression (i.e. benign, dysplastic, and invasive). We are also beginning to evaluate the mechanisms by which these transcripts are involved in prostate cancer progression. Identification of these genes and their transcripts as potential biomarkers of disease progression may lead to the development of novel diagnostic tools and less invasive and more efficacious targeted therapies.