Power production prediction of wind turbines using a fusion of MLP and ANFIS networks
Document Type
Article
Publication Date
7-9-2018
Publication Title
IET Renewable Power Generation
Volume
12
Issue
9
First Page
1025
Last Page
1033
Abstract
Access to accurate power production prediction of a wind turbine in future hours enables operators to detect possible underperformance and anomalies in advance. This may enable more proactive and strategic operations optimisation. This study examines common Supervisory Control And Data Acquisition (SCADA) data over a period of 20 months for 21 pitch regulated 2.3 MW turbines. In this study, an algorithm is proposed to impute values of data that are missing, out-of-range, or outliers. It is shown that an appropriate combination of a decision tree and mean value for imputation can improve the data analysis and prediction performance by the creation of a smoother dataset. In addition, principal component analysis is employed to extract parameters with power production influence based on all available signals in the SCADA data. Then, a new data fusion technique is applied, combining dynamic multilayer perceptron (MLP) and adaptive neuro-fuzzy inference system (ANFIS) networks to predict future performance of wind turbines. This prediction is made on a scale of one-hour intervals. This novel combination of feature extraction, imputation, and MLP/ANFIS fusion performs well with favourably low prediction error levels. Thus, such an approach may be a valuable tool for turbine power production prediction.
DOI
10.1049/iet-rpg.2017.0736
ISSN
17521416
E-ISSN
17521424
Recommended Citation
Morshedizadeh, Majid; Kordestani, Mojtaba; Carriveau, Rupp; Ting, David S.K.; and Saif, Mehrdad. (2018). Power production prediction of wind turbines using a fusion of MLP and ANFIS networks. IET Renewable Power Generation, 12 (9), 1025-1033.
https://scholar.uwindsor.ca/electricalengpub/265
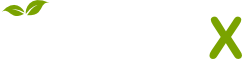
- Citations
- Citation Indexes: 45
- Usage
- Abstract Views: 2
- Captures
- Readers: 33
- Mentions
- References: 1