Constrained Generative Adversarial Learning for Dimensionality Reduction
Author ORCID Identifier
https://orcid.org/0000-0002-9956-4003
Document Type
Article
Publication Date
11-15-2021
Publication Title
IEEE Transactions on Knowledge and Data Engineering
Keywords
Generative adversarial networks, dimensionality reduction, deep learning, supervised learning, affinity correlation, separability constraint.
Abstract
Emerging data-driven technologies and big data analytics generate and deal with high-dimensional data. Transformation of such data into a low-dimensional feature space brings about numerous benefits, such as a more discriminant feature space, performance enhancement, less computational burden, and facilitating data visualization. This paper proposes a novel dimensionality reduction algorithm based on generative adversarial networks to tackle the issues related to high-dimensional data and common challenges in dimensionality reduction. To this aim, two constraints are defined to preserve the characteristics of the original data while rectifying the data distribution upon transformation. Formulating the transformation as sequential projections, the proposed Constrained Adversarial Dimensionality Reduction (CADR) method finds a set of sequential projection vectors that lead to a feature space in which between-class separability and within-class integrity are satisfied. This is while the transformed data perfectly comply with the pairwise affinity correlation in the original feature space. To evaluate the proposed method, nine advanced dimensionality reduction techniques are employed to enable a comparative study. The experiments are performed on several real-world benchmark datasets in terms of classification accuracy, F-measure, and G-mean. The obtained results show that the CADR could yield classification performance at a satisfactory level and outperforms the other competitors.
DOI
10.1109/TKDE.2021.3126642
Recommended Citation
Hallaji, Ehsan; Farajzadeh-Zanjani, Maryam; Razavi-Far, Roozbeh; Palade, Vasile; and Saif, Mehrdad. (2021). Constrained Generative Adversarial Learning for Dimensionality Reduction. IEEE Transactions on Knowledge and Data Engineering.
https://scholar.uwindsor.ca/electricalengpub/64
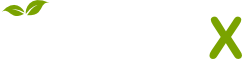
- Citations
- Citation Indexes: 9
- Usage
- Abstract Views: 14
- Captures
- Readers: 12
- Mentions
- News Mentions: 1