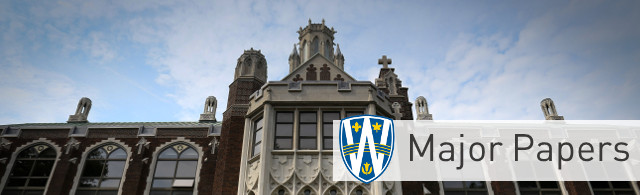
Abstract
Quantile regression seeks to extend classical least square regression by modeling quantiles of the conditional distribution of the response given the observed covariates. The attributes of quantile regression and its potential to handle different types of distributions, makes it possible to get rid of relying on normality assumptions and to solve problems in a more logical structure. It therefore provides crucial means to recognize effects that would not be noticed in classical least square regression. This study investigates Bayesian estimation of the 3-parameter generalized gamma distribution in the context of quantile regression, by allowing dependence of the model parameters on a covariate. The quantiles of the generalized gamma distribution are functions of the parameters, and in turn functions of the covariate. Our Bayesian estimation approach is compared to the maximum likelihood approach. Our work is validated via simulations to study the performance of the estimation methods. To demonstrate the use of the estimation methods, a data of corrosion is analyzed. Based on the Akaike Information Criterion (AIC) and/or Deviance Information Criterion (DIC), we infer that the model with interaction effects better fits the data.
Primary Advisor
Abdulkadir Hussein
Program Reader
Mohamed Belalia
Degree Name
Master of Science
Department
Mathematics and Statistics
Document Type
Major Research Paper
Convocation Year
2021