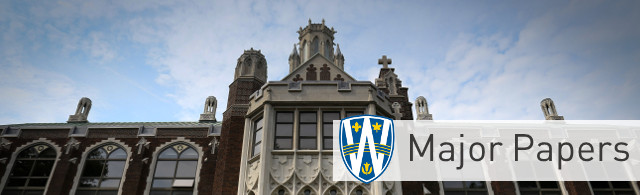
Abstract
Tensor data is widely used in modern data science. The interest lies in identifying and characterizing the relationship between tensor datasets and external covariates. These datasets, though, are often incomplete. An efficient nonconvex alternating updating algorithm proposed by J. Zhou et al. in the paper "Partially Observed Dynamic Tensor Response Regression" provides a novel approach. The algorithm handles the problem of unobserved entries by solving an optimization problem of a loss function under the low-rankness, sparsity, and fusion constraints. This analysis aims to understand in detail the proposed algorithms and their theoretical proofs with, potentially, dropping some of the assumptions implied to the model. Also, the efficiency and accuracy of the algorithms on a simulated data and Parkinson's disease real-life dataset will be illustrated.
Primary Advisor
Dr. Sévérien Nkurunziza
Program Reader
Dr. Abdul A. Hussein
Degree Name
Master of Science
Department
Mathematics and Statistics
Document Type
Major Research Paper
Convocation Year
2023